Bank Account Fraud: 5 Techniques Fraudsters Commonly Use
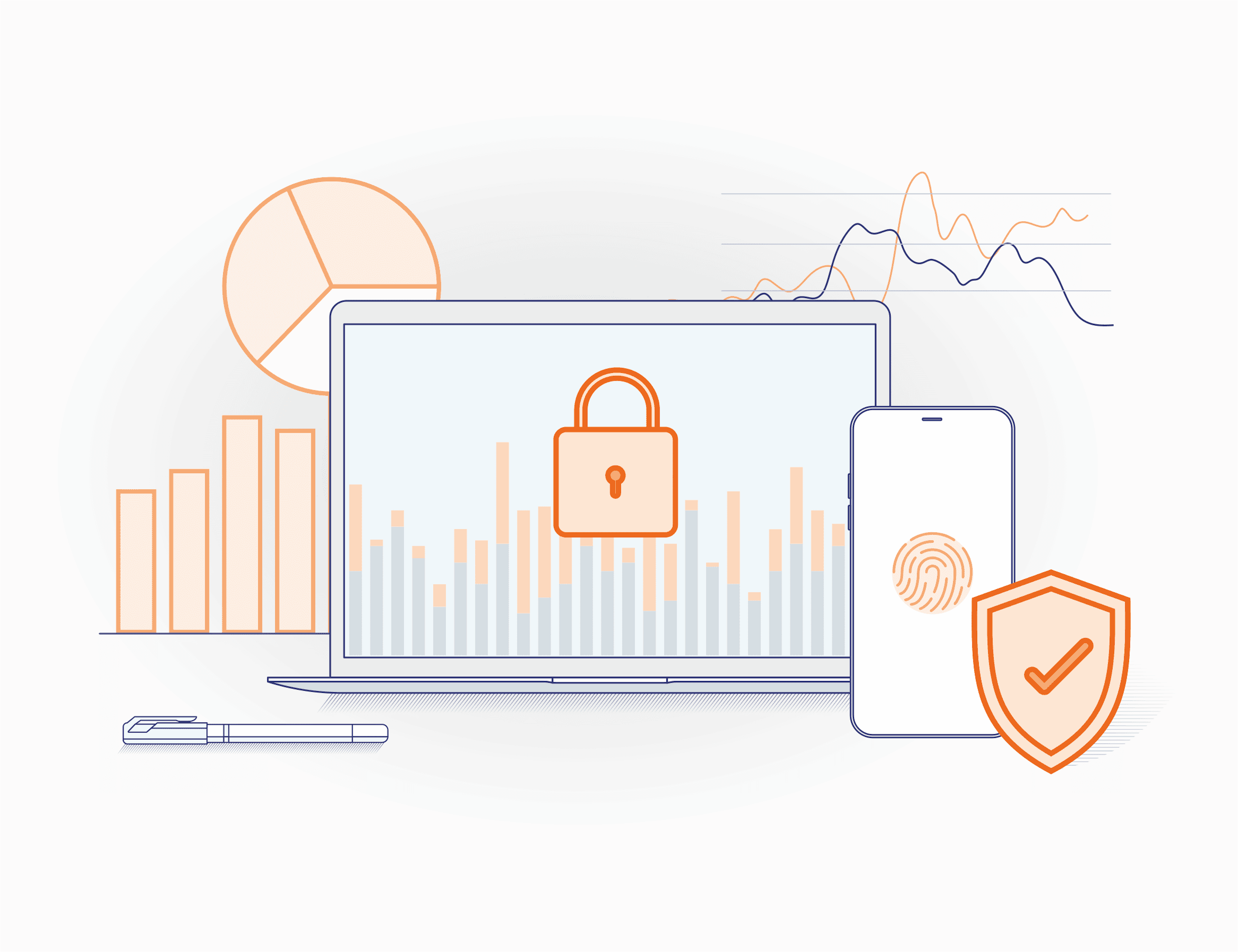
Bank account fraud is evolving fast – and so must our defenses.
According to Alloy's 2024 Financial Fraud Statistics study, financial institutions are experiencing a notable rise in fraudulent activities: more than half have noted a growth in fraud targeting business accounts, while over two-thirds have seen an uptick in consumer fraud cases. In 2023 alone, 35% of these institutions dealt with over 1,000 fraud attempts, and one in ten faced more than 10,000 incidents.
As financial services continue to digitize, fraudsters adapt just as quickly. From synthetic identities crafted by bots to unauthorized access through remote tools, today’s bank account fraud schemes are increasingly scalable and complex.
At JuicyScore, we support banks, fintechs, and digital lenders in over 30 countries. Our solutions help identify fraud risks that standard methods often overlook by analyzing behavioral and device-level signals – data points that reveal deeper patterns beyond traditional inputs.
This article provides an up-to-date overview of the five most common types of bank account fraud and explains how privacy-first fraud detection tools can help mitigate these risks.
Phishing remains one of the most prevalent entry points for bank account fraud. These attacks often rely on social engineering – deceiving users into revealing sensitive information or taking unauthorized actions. Tactics include spoofed emails, SMS phishing (smishing), fake websites, or fraudulent calls. In more sophisticated scenarios, deepfake voice messages or cloned banking app interfaces are used to deceive both customers and staff.
These fraud vectors often sit outside bank infrastructure, making them difficult to detect using internal controls alone. Addressing them effectively requires behavioral analysis and proactive anomaly detection.
In ATO fraud, attackers use stolen or compromised credentials to gain access to legitimate accounts. Once inside, they may transfer funds, apply for credit, or tamper with account details. Because the login appears valid, these actions often bypass traditional scoring models – if the credentials check out, the transaction may appear legitimate.
This is precisely why continuous monitoring of device consistency and behavioral anomalies is critical. A mismatch between historical and current device signals, signs of rooting, or the use of remote desktop software can reveal high-risk sessions that conventional tools overlook. Behavioral and technical signals provide an additional layer of intelligence – helping institutions detect suspicious activity before financial damage occurs.
Synthetic identity fraud occurs when real and fabricated data are combined to form a new identity. This could include a valid phone number and a fake name, paired with invented identification details. Fraudsters gradually build credit history, make regular repayments, and develop credibility before executing a large “bust-out” – disappearing with funds or loans.
What makes synthetic identity fraud particularly challenging is its long build-up period and surface-level legitimacy. It may go undetected until substantial losses occur.
JuicyScore helps identify signs of synthetic activity by analyzing randomized environments, emulators, virtual devices, and inconsistencies in behavioral interaction – insights that are often not available in standard data sets.
First-party fraud involves individuals who apply for financial products with no intention of repayment. This includes misstating income, hiding existing debts, or withdrawing funds before abandoning the account.
In markets with limited credit history or informal economies, first-party fraud can be difficult to distinguish from genuine thin-file customers. That’s where device intelligence, geolocation mismatches, and behavioral data provide valuable signals during the decision-making process.
Fraud rings often use “mule” accounts – bank accounts opened with stolen or synthetic identities – for laundering stolen funds. These accounts are used briefly to move money before being abandoned or shut down.
Mule detection requires pattern recognition at scale. Clusters of similar device fingerprints, short-lived accounts, or identical behavioral patterns across “different” customers are all red flags.
Probabilistic analysis of device environments helps identify subtle similarities that may indicate fraudulent activity, even in obfuscated sessions.
Bank account fraud is often invisible to systems that rely on historical financial data or static KYC documents. In markets with high informal income or thin credit files, this becomes even more pronounced.
What’s needed is a shift toward real-time, privacy-first insights that surface risk signals earlier – without collecting personal data.
To support institutions in detecting and mitigating complex fraud types, JuicyScore delivers over 220 non-personal signals based on device intelligence and behavioral analysis. This privacy-first approach enables stronger fraud defenses while ensuring compliance with global data regulations.
Let’s talk about how JuicyScore can strengthen your fraud prevention and credit risk modeling. Book your free demo with our team.
- Bank account fraud is growing in complexity.
- Synthetic identity fraud is the fastest-growing threat. Fraudsters build fake identities over time that appear legitimate – then vanish with loans or funds.
- Phishing and social engineering remain highly effective. Fraudsters trick users into giving up credentials using fake emails, websites, or messages – and even voice deepfakes.
- Account takeover (ATO) uses stolen credentials to access real accounts. Traditional systems often miss it unless device and behavior signals are checked.
- First-party fraud happens when customers themselves commit fraud – applying for products they never intend to repay. It’s common in thin-file or informal markets.
- Mule accounts are used by fraud rings to move stolen money. These often use fake or stolen identities and need network-level detection to uncover patterns.
- Traditional KYC and credit scoring models are no longer enough. Behavioral and device-based insights offer a privacy-safe way to detect fraud early and build stronger risk models.
- JuicyScore helps detect hidden fraud risks by analyzing 220+ device and behavior signals – without needing personal data.
Bank account fraud happens when someone illegally uses or manipulates a bank account to steal money, access financial services, or collect sensitive data. It includes scams like phishing, account takeovers, synthetic identity fraud, first-party fraud, and mule account activity.
Banks can spot fraud earlier by going beyond traditional credit data. Using behavioral patterns, device intelligence, and session analysis helps identify suspicious activity – even before money moves.
Watch for thin credit files that look too “clean,” mismatches between declared and real behavior, signs of virtual devices, or repeated patterns that suggest fake identities. These are strong red flags for synthetic identity fraud.
Social engineering tricks people into giving away passwords or security codes – often through fake emails or calls. Even with strong systems, human error caused by social engineering can open the door to serious fraud.
First-party fraud happens when someone uses their real identity to apply for credit – but never intends to repay. Unlike identity theft, it doesn’t involve impersonation, making it harder to detect with conventional tools.
Mule accounts are used to move or launder stolen funds. They’re often opened with fake or stolen data, used briefly, and then abandoned. Spotting them requires identifying shared behaviors or unusual device activity across accounts.
Old-school fraud tools rely on blacklists or credit history. But synthetic identities and social engineering don’t show up there. That’s why newer methods – like device fingerprinting and behavior analysis – are needed to spot fraud that looks “normal” on paper.
Synthetic identity fraud is on the rise. Discover how behavioral and device-based signals help fintechs detect synthetic fraud without using personal data.
Social engineering fraud types, impact on business, and ways to prevent.
Risk monitoring represents a set of techniques as a part of businesses’ risk-assessment.
Get a live session with our specialist who will show how your business can detect fraud attempts in real time.
Learn how unique device fingerprints help you link returning users and separate real customers from fraudsters.
Get insights into the main fraud tactics targeting your market — and see how to block them.
Phone:+971 50 371 9151
Email:sales@juicyscore.ai
Our dedicated experts will reach out to you promptly