What Is Alternative Credit Scoring and Why It Matters
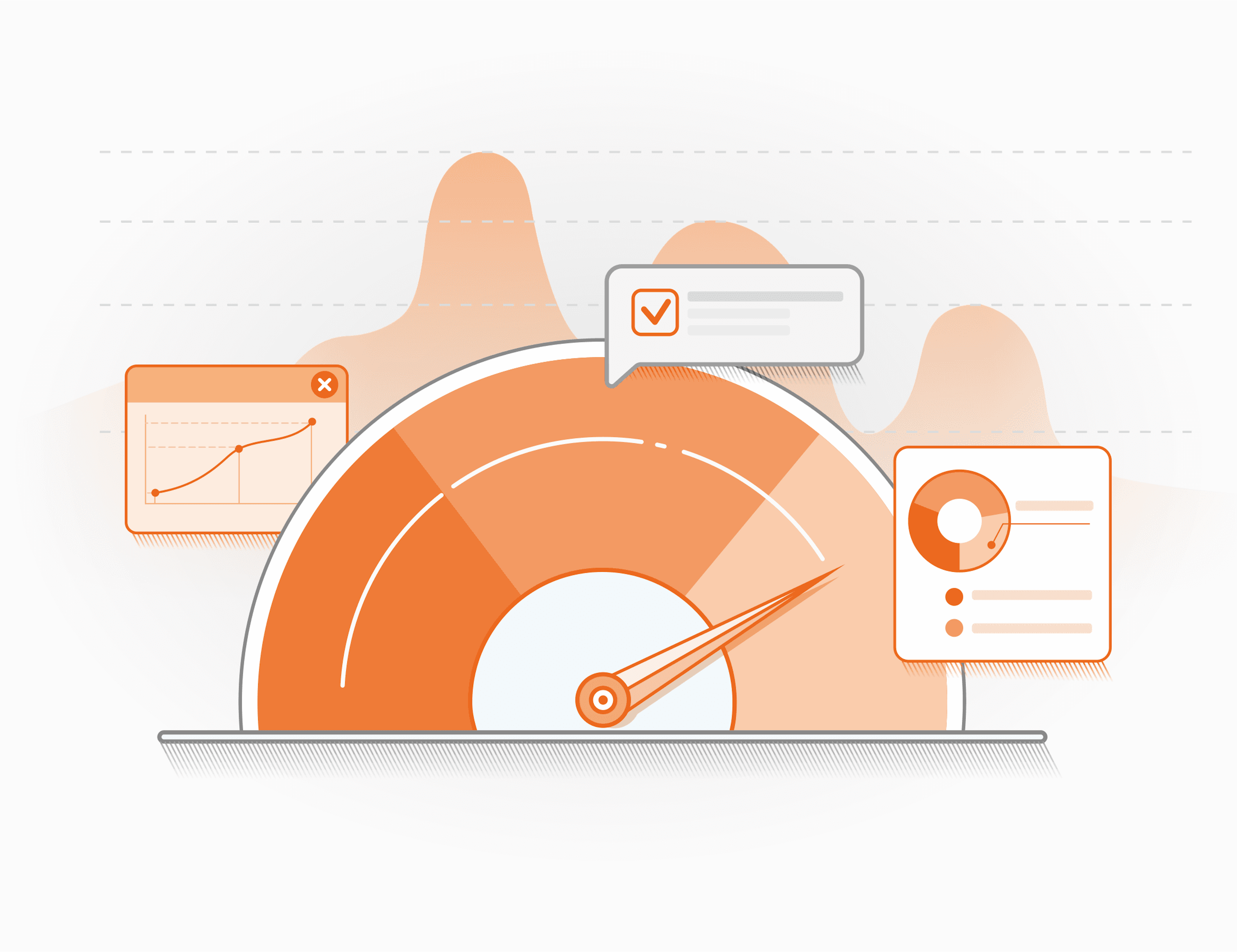
Alternative credit scoring is no longer a fringe concept – it’s becoming an essential tool for financial services looking to serve broader audiences, reduce risk, and operate with greater precision. Traditional credit data is often incomplete, outdated, or unavailable – especially in emerging markets. As digital lending continues to grow, so does the need for smarter, more adaptive ways to assess risk.
At JuicyScore, we’ve seen how non-personal, behavioral and device-based signals can strengthen decision-making without compromising privacy. In this article, we’ll walk through what alternative credit scoring actually is, how it works, and why it matters right now.
Credit bureaus rely on historical data: repayment history, loan balances, and credit inquiries. While useful, this system leaves out a huge portion of the global population – those who are new to credit, earn informal income, or simply don’t have enough financial history to generate a reliable score. This especially applies to emerging markets like Kenya, Nigeria, Indonesia, or the Philippines, where informal income is common and credit penetration is low.
This gap creates friction for both lenders and customers. It leads to missed opportunities and higher default rates. It also makes expansion into underbanked markets riskier and more expensive than it needs to be.
1.4 billion people in the world remain unbanked, according to the World Bank Group’s Global Findex 2021 database. Alternative credit scoring can work for the unbanked population – and be profitable to financial institutions, says Islam Zekry, Chief Data Officer at Commercial International Bank (Egypt). He points out:
But it isn’t just about financial access – it’s also about the role that alternative credit scoring can play in preventing fraud. Many digital lenders are facing attacks that are harder to detect with standard tools.
For example, in Africa fraudulent access to digital loans through fintech platforms has been steadily increasing. Chris Akolo, Regional Business Development Director for Kenyan market at JuicyScore, notes:
Alternative credit scoring refers to the use of non-traditional data sources to assess creditworthiness. These sources might include:
Instead of relying solely on a customer’s past loans or financial statements, alternative credit scoring tools analyze real-time behavioral data. For example:
This type of alternative data for credit scoring not only improves fraud detection but also helps financial institutions better understand risk in thin-file or no-file customers – a common case in emerging markets.
For example, JuicyScore’s solution provides over 220 parameters derived from device intelligence and user behavior, all without collecting personal data. This allows lenders to get valuable inputs for credit risk modeling, enabling more robust and data-driven scoring systems. JuicyScore’s Chris Akolo explains:
In many countries, regulatory pressure around the use of personal data is increasing rapidly. Governments are tightening data protection rules, requiring more disclosures, and limiting how financial institutions collect, store, and process sensitive customer information.
Frameworks like the EU’s General Data Protection Regulation (GDPR), Brazil’s Lei Geral de Proteção de Dados (LGPD), and Nigeria’s Data Protection Regulation (NDPR) have set new global benchmarks for privacy compliance.
And other countries are following suit. For example, in 2022 Indonesia has passed Personal Data Protection Law (PDP Law) – a major milestone for data privacy in Southeast Asia. Often referred to as Indonesia’s version of the GDPR, the law introduces strict requirements for obtaining user consent, data minimization, and the clear specification of processing purposes. Organizations must also provide accessible and transparent privacy notices – including on websites and landing pages – to inform users about how their data is being used.
Tech giants are also strengthening their stance on data privacy.
For example, in 2023 Google announced a significant update to its policy for personal loan apps listed on the Google Play Store, restricting these apps from accessing sensitive permissions on Android devices. Apps involved in digital lending or personal loan facilitation are now prohibited from accessing data such as user contacts, photos, location, call logs, or external storage.
This move is part of a broader industry trend where platform providers are placing stricter controls on how apps collect and handle user data. Apple has taken similar steps through its App Tracking Transparency (ATT) framework, and browsers like Firefox and Safari have implemented features to block third-party tracking by default.
This shift creates serious friction for lenders and fintech companies that rely on traditional credit data – especially when that data includes personally identifiable information (PII). Handling PII means added legal risk, compliance overhead, and often delays in decision-making.
A privacy-first approach to credit risk assessment removes much of that burden. By using anonymized behavioral and device-based signals instead of personal data, alternative credit scoring helps lenders stay compliant, agile, and competitive – even in jurisdictions with strict regulations.
Today’s financial landscape is changing fast. Privacy regulations are tightening. Borrowers expect greater transparency. And competitive advantage now depends on how quickly – and accurately – you can assess risk without relying on sensitive personal data.
What many institutions still lack is a reliable way to score credit when traditional data falls short. That’s where alternative credit scoring comes in.
Whether you're a bank entering new markets, a BNPL provider optimizing approvals, or a neobank managing risk across new segments – this layer of behavioral and device-based insight could be exactly what your credit model is missing.
JuicyScore’s clients have seen outstanding results using our solutions – with an average ROI of 10X+ and a GINI coefficient of 5.20+.
If you’d like to see how alternative credit scoring can work for your business – consider booking a free demo with the JuicyScore team. We’ll provide a personalized consultation, walk you through the product, and show how it can fit into your existing credit decisioning process. Book your demo here.
- Traditional credit data is limited, especially in emerging markets where informal income and thin-file borrowers are common.
- Alternative credit scoring uses non-traditional data — such as device behavior, network signals, and user activity — to assess creditworthiness in real time.
- Alternative credit data is a powerful input for credit risk modeling, enabling more accurate, adaptable, and predictive scoring systems.
- Alternative credit scoring can use anonymized behavioral and device signals instead of personal data.
- Using non-personal data helps reduce legal risk, accelerate decision-making, and maintain compliance in increasingly regulated digital environments.
Alternative credit scoring is a method of assessing a borrower's creditworthiness using non-traditional data. This includes behavioral patterns, device integrity, browser configuration, and online activity instead of relying on financial history or credit bureau records.
It’s especially useful in regions with low credit penetration or informal economies, where traditional scores often fall short.
An alternative credit score is calculated using data like device behavior, connection quality, network stability, and signs of spoofing or remote access tools. These data points are used to create a risk profile in real time — helping lenders make informed decisions even when applicants have no formal credit history.
At JuicyScore, we use over 220 parameters, all without collecting personal data.
The key difference is the type of data used.
This makes it far more inclusive and responsive in digital lending contexts.
When combined with predictive modeling, alternative data for credit scoring can offer strong accuracy and early fraud detection.
Behavioral and device signals are harder to fake than traditional identity documents and can flag high-risk patterns like synthetic identities, bot usage, or remote control attempts before they cause losses.
Alternative credit scoring provides behavioral and device-based signals that serve as valuable inputs for credit risk modeling. These data points help build more flexible, accurate, and responsive models, especially in markets where traditional credit history is incomplete or unavailable.
Alternative credit scoring systems typically rely on anonymized, non-personal data such as device characteristics, behavioral patterns, and network signals. By avoiding the use of personally identifiable information (PII), these systems are better aligned with modern data privacy regulations.
Understanding the role of behavioral biometrics in fraud prevention.
Risk scoring factors plays a vital role in fraud detection and prevention strategies.
Synthetic identity fraud is on the rise. Discover how behavioral and device-based signals help fintechs detect synthetic fraud without using personal data.
Get a live session with our specialist who will show how your business can detect fraud attempts in real time.
Learn how unique device fingerprints help you link returning users and separate real customers from fraudsters.
Get insights into the main fraud tactics targeting your market — and see how to block them.
Phone:+971 50 371 9151
Email:sales@juicyscore.ai
Our dedicated experts will reach out to you promptly